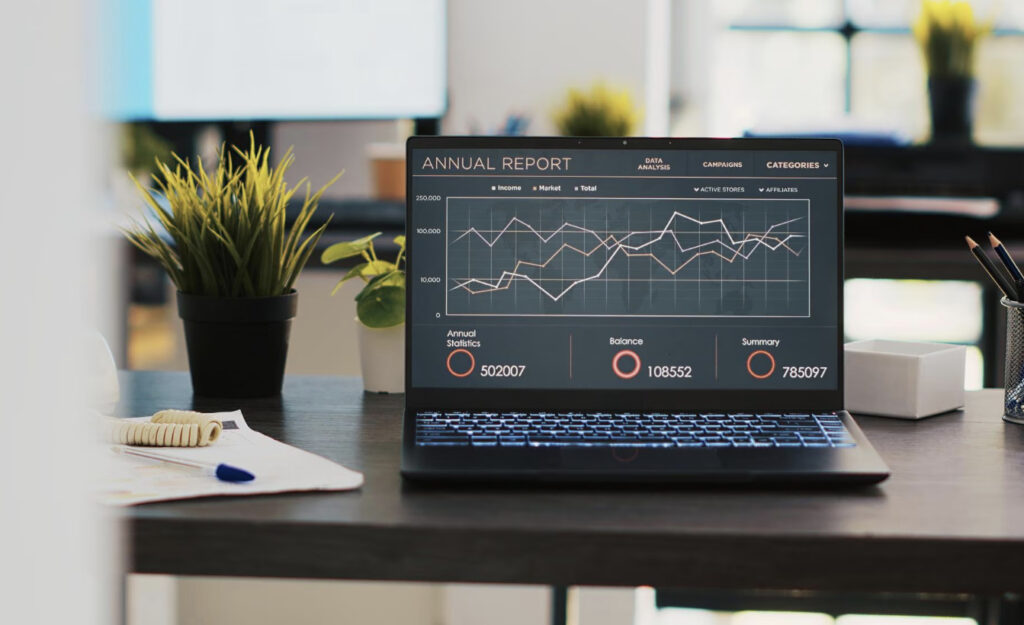
In today’s data-driven world, sales forecasting is more critical than ever. Businesses can no longer rely on guesswork or outdated methods to predict future sales. This is where predictive analytics comes in. By leveraging machine learning and advanced algorithms, predictive analytics allows companies to analyze large datasets, identify trends, and make more informed decisions regarding their sales strategies. Integrating this technology into your sales process provides greater accuracy, efficiency, and foresight into market demands. In this article, we will explore how predictive analytics is transforming sales forecasting, its key benefits, and best practices for implementation.
What is Predictive Analytics in Sales Forecasting?
Predictive analytics is a data-driven approach that uses statistical algorithms and machine learning techniques to analyze historical data and forecast future outcomes. When applied to sales forecasting, it evaluates past sales patterns and external factors such as market trends, customer behavior, and seasonal fluctuations to predict future sales performance more accurately.
Traditional sales forecasting methods typically focus on past sales figures and business intuition. While these methods provide a general outlook, they often fail to account for variables that impact sales, such as changing customer preferences or economic shifts. Predictive analytics, on the other hand, allows companies to incorporate real-time data and external influences, leading to more accurate forecasts.
By adopting predictive analytics, businesses can understand what drives their sales, anticipate demand more effectively, and make data-backed decisions. As a result, companies can align their resources more closely with actual market conditions, reducing the risks of stock shortages, overproduction, or missed opportunities.
How Predictive Analytics Transforms Traditional Sales Forecasting
Traditional sales forecasting methods often rely on historical sales data, market assumptions, and business experience. These methods, while helpful, are limited by their reactive nature. They focus on what happened in the past rather than considering current market dynamics or future trends.
Predictive analytics transforms this process by using data science and machine learning to incorporate multiple data streams, including customer interactions, economic indicators, and external market conditions. This broader data scope enables businesses to anticipate not only sales but also shifts in demand, customer preferences, and market conditions.
For instance, if a company relies solely on historical sales data, it may not anticipate a sudden spike in demand caused by an external event, such as a new product release by a competitor or an economic boom. Predictive analytics can capture such patterns in real-time, allowing businesses to adjust their sales strategies accordingly. This proactive approach helps companies respond quickly to changes, ultimately leading to better sales outcomes and reduced forecast errors.
Predictive analytics also helps businesses avoid costly mistakes, such as overstocking or understocking. By using real-time data and machine learning models, companies can predict future demand more accurately, leading to optimized resource allocation and reduced waste.
Key Benefits of Predictive Analytics for Sales Forecasting
Predictive analytics brings numerous advantages to the sales forecasting process, helping businesses make smarter decisions and stay ahead of the competition.
Improved Accuracy in Sales Predictions
One of the primary benefits of predictive analytics is improved forecast accuracy. Traditional sales forecasting methods often rely on limited datasets and historical trends, which may not fully reflect future sales potential. Predictive analytics, by contrast, leverages large datasets, including customer behavior, market conditions, and social trends, to create more comprehensive sales predictions.
This data-driven approach reduces the margin of error in sales forecasts and helps businesses make more precise decisions about inventory, marketing, and resource allocation. Companies that use predictive analytics can anticipate changes in demand more effectively and adjust their strategies in real-time, avoiding issues like stock shortages or excess inventory.
Optimized Inventory Management
Accurate sales forecasting through predictive analytics leads to better inventory management. With a clearer view of future demand, businesses can optimize stock levels to avoid both overproduction and stockouts. Predictive models provide insights into sales trends, helping companies maintain the right amount of inventory at the right time.
This optimization is particularly beneficial during peak sales periods or for seasonal products, where misjudging demand can lead to significant financial losses. By aligning inventory with demand forecasts, companies can lower operational costs, improve cash flow, and enhance customer satisfaction.
Building an Effective Predictive Sales Model
To fully leverage predictive analytics in sales forecasting, businesses need to develop a robust predictive model. This involves several key steps:
- Data Collection: Gather relevant data from various sources, such as CRM systems, sales records, market trends, and customer interactions. Ensure that the data is clean, up-to-date, and comprehensive.
- Algorithm Selection: Choose the appropriate machine learning algorithms to process the data. Commonly used algorithms for sales forecasting include regression analysis, decision trees, and neural networks. The choice of algorithm depends on the complexity of your sales data and your specific forecasting needs.
- Model Validation: After building the predictive model, it’s essential to test and validate its performance. Regularly review and adjust the model to ensure it continues to provide accurate forecasts as new data becomes available. This step is crucial for adapting to changing market conditions and improving the model’s reliability over time.
By following these steps, companies can build a predictive model that enhances the accuracy and efficiency of their sales forecasting efforts.
Best Practices for Implementing Predictive Analytics for Sales Forecasting
Successful implementation of predictive analytics in sales forecasting requires adherence to several best practices. These steps ensure that your forecasts are accurate and actionable.
Data Quality and Integrity
The foundation of any predictive analytics model is the quality of the data it uses. Inaccurate or incomplete data can lead to flawed forecasts, reducing the model’s effectiveness. To ensure data integrity, regularly clean and update your datasets. This involves removing duplicates, correcting errors, and filling in missing information. The better the quality of your data, the more reliable your sales forecasting results will be.
Choosing the Right Predictive Tools
Selecting the right predictive analytics tools is critical for effective sales forecasting. There are various software solutions available, each offering different features such as scalability, user-friendliness, and integration with existing systems. When choosing a tool, consider factors such as your business size, data complexity, and budget. Popular tools include IBM Watson, Salesforce Einstein, and Microsoft Azure Machine Learning.
The right tools not only make it easier to gather and analyze data but also allow for better collaboration between sales teams and other departments, ensuring everyone is aligned on forecasting goals.
Future Trends in Predictive Analytics for Sales Forecasting
As technology continues to advance, predictive analytics will play an even larger role in sales forecasting. New developments in artificial intelligence (AI) and machine learning are enabling businesses to create even more precise sales models.
One emerging trend is the use of real-time data feeds, which provide companies with up-to-the-minute insights into market conditions and customer behavior. This allows businesses to adjust their sales strategies instantly, ensuring they stay competitive in a rapidly changing environment.
Another trend is the increasing accessibility of predictive analytics tools. As more user-friendly platforms become available, even small and medium-sized businesses will be able to harness the power of predictive analytics for sales forecasting, leveling the playing field with larger competitors.
In the future, we can also expect greater integration between predictive analytics and other business functions, such as marketing and customer service. This will allow for more comprehensive business planning and a more holistic approach to meeting customer needs.
Predictive analytics is revolutionizing sales forecasting by offering a more accurate, data-driven approach. Companies that embrace this technology can improve sales predictions, optimize inventory, and make smarter business decisions. By following best practices and staying ahead of emerging trends, businesses can fully leverage predictive analytics to drive long-term growth and success.